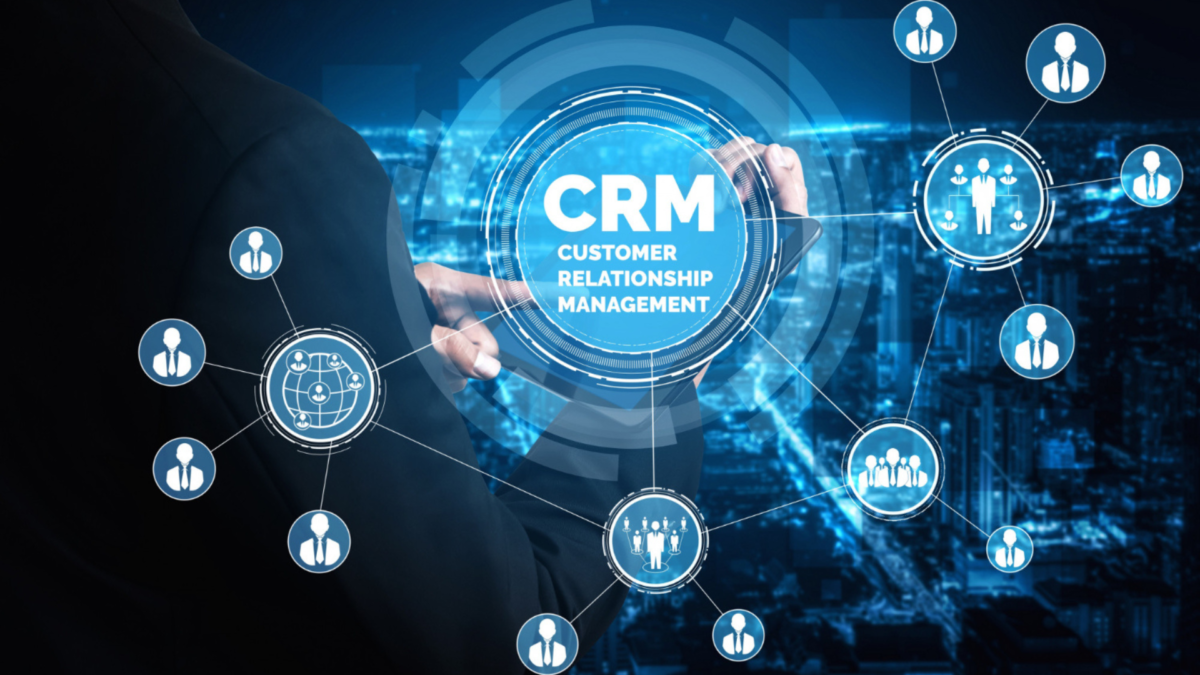
customer relationships are at the heart of success. Managing these relationships effectively is crucial, and that’s where CRM (Customer Relationship Management) systems come into play. Over the years, CRM systems have evolved, offering much more than just a way to manage customer information. With the integration of advanced technologies like predictive analytics, CRM systems are becoming smarter, more efficient, and better equipped to help businesses foster long-term customer loyalty.
In this article, we’ll explore the role of predictive analytics in CRM development and how businesses can benefit from integrating this technology to enhance customer engagement, improve decision-making, and drive growth.
What is Predictive Analytics in CRM?
Predictive analytics in CRM refers to using historical data, statistical algorithms, and machine learning techniques to predict future customer behavior. It involves analyzing patterns in customer data and making predictions about future outcomes, such as customer purchasing behavior, churn rates, or potential sales opportunities.
Integrating predictive analytics into CRM systems allows businesses to make data-driven decisions, optimize their marketing and sales strategies, and enhance overall customer experiences. By leveraging predictive insights, businesses can stay ahead of trends and act proactively to meet customer needs.
Click to Explore the Best App Development Company in USA.
The Benefits of Integrating Predictive Analytics in CRM Development
Integrating predictive analytics into CRM systems can provide businesses with a competitive edge by helping them identify opportunities and mitigate risks before they arise. Here are the key benefits:
1. Enhanced Customer Segmentation
One of the most significant advantages of predictive analytics is its ability to segment customers based on their behavior, needs, and preferences. By analyzing past interactions, purchasing patterns, and demographics, businesses can categorize customers into specific groups, such as:
- High-Value Customers: Customers who are more likely to make repeat purchases or have high lifetime value.
- At-Risk Customers: Customers who show signs of disengagement or may be at risk of churning.
- Prospective Buyers: Customers who are likely to convert in the near future.
This level of segmentation allows businesses to tailor their marketing, sales, and customer service efforts to meet the specific needs of each group, ultimately improving customer satisfaction and driving higher conversion rates.
2. Predicting Customer Behavior
Predictive analytics enables businesses to forecast future customer behavior, such as purchase likelihood, product preferences, and buying frequency. For example, by analyzing a customer’s purchase history, a predictive CRM system can suggest when they are likely to make their next purchase or what products they may be interested in.
This capability helps businesses personalize their offerings and messaging, increasing the chances of engaging customers at the right time with the right products. Personalized recommendations lead to improved customer experiences and higher sales conversion rates.
3. Improved Lead Scoring and Sales Forecasting
Predictive analytics helps sales teams focus their efforts on the leads that are most likely to convert. By analyzing data such as lead behavior, engagement history, and demographic information, CRM systems can assign a score to each lead, indicating the probability of conversion. This allows sales teams to prioritize high-potential leads, reducing wasted time on unqualified prospects.
Additionally, predictive analytics helps businesses forecast future sales more accurately. By analyzing past sales data and trends, businesses can predict future revenue and set more accurate sales goals. This can help optimize resource allocation, production planning, and marketing strategies.
4. Reducing Customer Churn
One of the most significant challenges businesses face is customer churn—the loss of customers who stop doing business with them. Predictive analytics helps identify early warning signs of churn, such as reduced engagement, slower response times, or declining purchase frequency. By identifying these at-risk customers, businesses can take proactive steps to retain them.
For example, predictive CRM systems can trigger automated alerts for account managers to reach out to at-risk customers with personalized offers or special discounts to prevent churn. By addressing churn before it happens, businesses can improve retention rates and maintain a loyal customer base.
5. Optimizing Marketing Campaigns
Predictive analytics can help businesses optimize their marketing campaigns by identifying which strategies are most likely to yield positive results. By analyzing historical campaign data, businesses can predict which types of content, messaging, and channels will resonate most with specific customer segments.
Additionally, predictive analytics can help businesses identify the ideal timing for campaigns. For example, if predictive models reveal that certain customers are more likely to engage with an email campaign during specific months or seasons, businesses can time their outreach accordingly for maximum impact.
Bonus Blog: Top-Rated App Development Companies in the USA: A Comprehensive Guide
How Predictive Analytics Works in CRM Systems
The integration of predictive analytics into CRM systems involves several key steps:
1. Data Collection and Integration
The first step in integrating predictive analytics into CRM systems is collecting and integrating data from various sources, including:
- Customer interactions (emails, calls, chats)
- Social media activity
- Purchase history
- Website visits and behavior
- Customer feedback
This data forms the foundation for predictive models, as it provides the raw information needed to analyze trends and behaviors.
2. Data Processing and Analysis
Once the data is collected, it is processed and analyzed using statistical algorithms and machine learning models. These models identify patterns in the data that are indicative of future customer behavior.
For example, a machine learning algorithm might analyze a customer’s previous purchase behavior and determine that they are likely to purchase a specific product within the next month. This insight allows businesses to take targeted actions, such as sending a personalized offer or reminder email.
3. Model Training and Refinement
To ensure the accuracy of predictions, predictive models must be trained and refined over time. As more data is collected, the models are continuously updated to improve the accuracy of future predictions. This ongoing learning process helps businesses adapt to changing customer behaviors and market trends.
4. Actionable Insights
Finally, predictive analytics delivers actionable insights that businesses can use to optimize their CRM strategies. These insights can be presented in the form of dashboards, reports, or alerts, allowing businesses to take quick action based on real-time data.
Choosing the Right Custom CRM Development Company
When it comes to integrating predictive analytics into CRM systems, partnering with the right custom CRM software development company is essential. A specialized CRM development company has the expertise to design and implement predictive models that align with your business objectives. They can help you:
- Customize Predictive Analytics Models: Build tailored predictive models based on your unique customer data and business needs.
- Integrate with Existing Systems: Ensure seamless integration of predictive analytics tools into your existing CRM system.
- Optimize CRM Performance: Continuously optimize the performance of predictive models to improve the accuracy of predictions and enhance CRM effectiveness.
By partnering with a custom CRM development company that specializes in predictive analytics, businesses can unlock the full potential of their CRM systems and gain a competitive edge in customer relationship management.
Conclusion
Predictive analytics is transforming the CRM landscape, enabling businesses to anticipate customer needs, optimize marketing strategies, and improve customer retention. By integrating predictive analytics into CRM systems, businesses can gain valuable insights into customer behavior, enhance segmentation, and make data-driven decisions that drive growth.